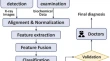
Overview
Health Information Science and Systems is a multidisciplinary journal that integrates computer science/information technology with health science and services, embracing information science research coupled with topics related to the modeling, design, development, integration, and management of health information systems.
The scope includes:
- Medical/health/biomedicine information resources, such as patient medical records, devices and equipments, software and tools to capture, store, retrieve, process, analyze, optimize the use of information in the health domain,
- Data management, data mining, and knowledge discovery, all of which play a key role in decision making, management of public health, examination of standards, privacy and security issues,
- Development of new architectures and applications for health information systems.
- Editor-in-Chief
-
- Yanchun Zhang
- Impact factor
- 6.0 (2022)
- 5 year impact factor
- 5.7 (2022)
- Submission to first decision (median)
- 21 days
- Downloads
- 200,039 (2023)
Latest articles
Journal updates
-
Meet the Editors of Health Information Science and Systems
Professor Yanchun Zhang (Editor-in-Chief), Zhejiang Normal University & Peng Cheng Laboratory, China; Victoria University, Australia
Learn more about Prof Zhang.Dr. Siuly Siuly (Managing Editor), Victoria University, Australia
Learn more about Dr. Siuly. -
Call for Papers: Special Issue on COVID-19
Guest editors: Siuly Siuly, Guanzhen Yu
-
Call for Papers: Special Issue in Data Science Approaches to Innovative Aging Management and Care
Guest editors:
Dr. Qingpeng Zhang, Dr. Haolin Wang, Dr. Xiaoying Tang, Dr. Mingyang Li, Dr. Zoie WongDeadline of submission: February 28, 2022
-
Call for Papers: Special Issue in Integrative Multi-omics for Human Health and Disease Studies
Guest editors:
Dr. Yunpeng Cai, Dr. Yaohang Li, Dr. Xuan Guo, Dr. Eric Lu ZhangDeadline of Submission: May 31, 2023
Journal information
- Electronic ISSN
- 2047-2501
- Abstracted and indexed in
-
- Baidu
- CLOCKSS
- CNKI
- CNPIEC
- Current Contents/Clinical Medicine
- DBLP
- Dimensions
- EBSCO
- EMBASE
- Gale
- Google Scholar
- Japanese Science and Technology Agency (JST)
- Naver
- Norwegian Register for Scientific Journals and Series
- OCLC WorldCat Discovery Service
- Portico
- ProQuest
- PubMedCentral
- Reaxys
- SCImago
- SCOPUS
- Science Citation Index Expanded (SCIE)
- TD Net Discovery Service
- Wanfang
- Copyright information